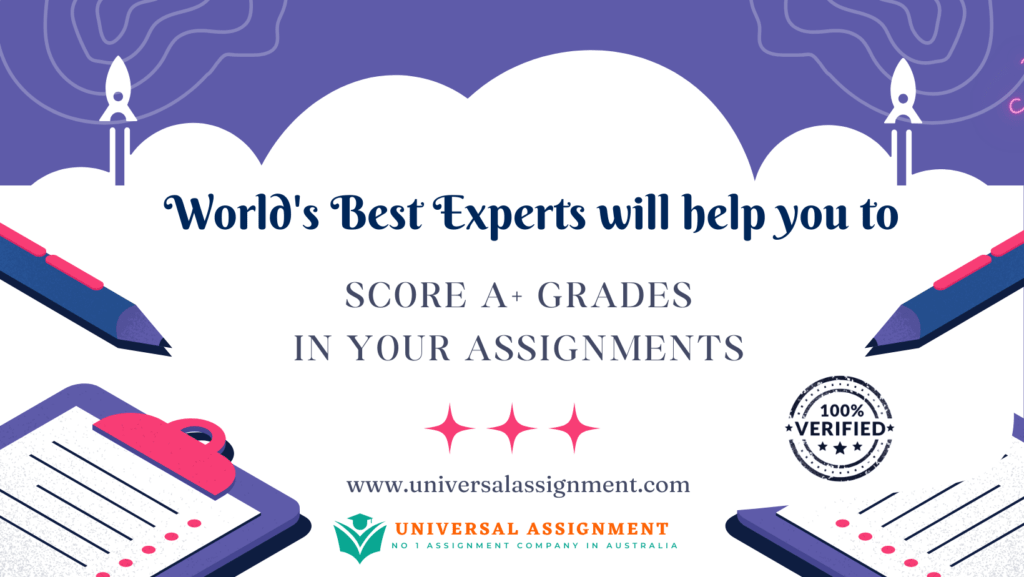
Lung blood clot detection is a critical task for clinicians, as early diagnosis and treatment can prevent potentially fatal outcomes. However, the task is difficult, as pulmonary embolism (a blood clot in the lungs) can be difficult to diagnose, particularly in its early stages. The current standard of care for detecting blood clots in the lung is a CT scan, which is expensive and exposes the patient to radiation (Bĕlohlávek, Dytrych & Linhart, 2013).
Recent advances in deep learning have shown promise for automated detection of a wide range of medical conditions (Farhat, Sakr & Kilany, 2020). In this study, we seek to apply deep learning to the task of detecting blood clots in the lungs.
The main components of blood include plasma, red blood cells (RBC), white blood cells (WBC) and platelets. Plasma is a clear colourless fluid that contains water and other substances, and comprises about 55 percent of blood volume. It contains many vital life functions such as transport of oxygen in the body, blood clotting capability and hormonal regulation and, responsible for blood clotting (Batalis & Harley, 2013).
Blood clots are one in all the most important reasons of stroke and coronary heart attack. Blood can become trapped in sticky blood vessels and block blood flow. This results in a life-threatening situation if blood clots block your brain or heart causing a stroke or heart attack. Blood clotting can start at any age but usually happens in your 20s and 30s. Signs and symptoms include: headache, sudden pain in the chest, arm or leg, numbness or tingling in one or more parts of the body, rapid heartbeat/heart palpitations and shortness of breath (Bĕlohlávek, Dytrych & Linhart, 2013).
There are a variety of diagnostic techniques that physicians can use when a blood clot is suspected. Certain tests use imaging tools such duplex ultrasound, magnetic resonance imaging (MRI), venography, computed tomography scans, magnetic resonance angiography, D-Dimer test, arteriography/angiography, and impedance plethysmography (Batalis & Harley, 2013).
These techniques differ in the methods they use to determine the presence of blood clots and are also specifically designed to detect various medical conditions. They are very expensive, inaccurate and prone to delayed diagnosis, so not all laboratories have them available.
Many researches were studied to detect blood clots in early stages using neural network models, genetic algorithms and Artificial Intelligence (AI). Deep learning detection of blood clots in the lungs works by training a deep neural network to identify blood clots in lungs. The network is trained with a set of images. Once trained, the network can easily identify blood clots in new images, based on their shape and location. Deep learning is a very exciting area of computer science with many applications in the real world. It has already shown power in many application fields, and has great potential to improve the overall performance of machine learning systems (Farhat, Sakr & Kilany, 2020). Therefore, in this research, we will use deep learning methods to predict blood clots in lungs.
1.1 Research Aim and Objectives
Blood clots in the lung can have devastating consequences. There is no easy or reliable way to detect them, so the best way to avoid problems is to prevent them. This project aims to develop a machine learning model to perform quick and accurate blood clot detection in lungs.
The overall purpose of this project is to apply deep learning in order to detect blood clots in lungs. This can save lives of people who have pulmonary embolism, a potentially fatal condition that involves clots in the arteries of the lung.
The following objectives will be accomplish to achieve the general objective of
the study.
- To conduct comprehensive systematic literature review so as to identify methods, algorithms and approaches used in this study.
- To label the data by experts.
- To prepare training and test dataset.
- To identify suitable deep learning algorithms.
- To develop an optimal model to detect blood clot in lungs.
- To test and evaluate the performance of the proposed model.
1.2 Scope and Limitations
This study will only focus on developing a deep learning model to detect blood clots in the lungs. Deep learning algorithms are powerful tools that can be used to detect and diagnose a wide range of conditions, including blood clots. These algorithms can detect blood clots more accurately and quickly than traditional methods, and they can be used to detect and diagnose conditions even in early stages.
Additionally, deep learning algorithms are more cost-effective and require less manual labor than traditional methods. However, it has also certain limitations to using them to detect blood clots. For example, deep learning algorithms require large amounts of data for training and may not be able to detect rare conditions or conditions in early stages. Additionally, the accuracy of deep learning algorithms can vary depending on the data used for training and the quality of the images used for analysis.
Chapter 2: Literature Review
2.1 Background Overview
Pulmonary embolism (PE) refers to blood clots in the pulmonary arterial system of the lungs, which typically originate in the deep veins of the legs and move to the lung’s blood vessels, where they become trapped. PE results in decreased blood flow and oxygen levels to the lungs as well as to other organs in the body. PE is linked to substantial morbidity and mortality (Bĕlohlávek, Dytrych & Linhart, 2013).
Multiple risk factors, including immobilisation, recent surgery, a history of clotting disorders, malignancy, obesity, pregnancy, cigarette smoking, certain medications such as birth control pills, and medical conditions such as heart disease, predispose patients to the development of pulmonary embolism (PE) (Batalis & Harley, 2013). Early diagnosis and treatment can significantly lower the chance of mortality. Consequently, correct diagnosis is essential for these patients (Brevik, 2019).
In this aspect, Deep Learning can assist in predicting the existence of PE by emphasising regions of interest with varied degrees of certainty, resulting in a quicker and more precise diagnosis for the patient. This instrument can assist in identifying life-threatening PEs, particularly those that are acute and central. It is crucial to detect PEs early because they are associated with a greater death rate. This method can also assist in ruling out PE and various subtypes of existent PE, allowing radiologists to prioritise tests and triage patient management (Farhat, Sakr & Kilany, 2020).
2.2 Overview of Deep Learning
AI is a general word that covers a wide range of techniques. Based on neural networks, deep learning is a branch of artificial intelligence. These artificial networks are made up of many interconnected layers of neurons. In essence, each neuron consists of a single linear regression unit. Each neuron receives its input from the neurons in the layer above. “Weights” refers to the connections between the neurons. comparing and contrasting synthetic and biological neural networks. There are numerous interconnected layers that make up neural networks. The network receives data, and an output is created. An error can be calculated by comparing the network’s output to the desired true label. The method optimizes connections between the layers based on the error. “Weights” refers to the connections between the neurons. A tuned network is eventually achieved.
Input data is supplied into the network during training, and the result is computed. Error estimation is possible because of the discrepancy between the estimated label, which is the network output, and the genuine label. The algorithm can adjust the weights of the network to optimize it by estimating the error of the model output. Backpropagation is the name of this network optimization procedure. Important network connections are strengthened and irrelevant connections are blocked by adjusting the weights. In this method, the inaccuracy of the network is reduced and the discrepancy between the network outputs and the genuine labels is minimized.
2.3 Neural Networks with Convolutions
The cornerstone deep learning networks for image processing are convolutional neural networks (CNN). According to (Müller & Kramer, 2021) CNNs were created specifically to process images. There are numerous filters in each CNN layer (Guo et al., 2018). Similar to the weights of general neural networks, each filter is a tiny matrix of weights. Pixels in the image are subjected to the filters repeatedly. The filters notice recurring patterns since they are applied to the entire image. CNNs are therefore perfect for analyzing photos because they are made up of recurring patterns. Lines, circles, and other basic geometric patterns are recognized by CNN’s shallow layers as low-level patterns. A high-level comprehension of the image, such as context (i.e., “image with PE” vs. “image without PE”), is gained via the deeper layers. Medical image analysis has seen a significant revolution thanks to CNN in recent years (Shen et al., 2017). Convolutional neural network architecture (CNN). CNNs are networks created specifically for image processing. Each CNN layer is made up of many tiny filters. The image pixels are subjected to a series of applications of a tiny matrix of weights known as a filter. Recurring patterns are identified by applying the filter to the entire image. Since images are made up of recurring patterns, CNNs are excellent for image analysis. Low-level patterns are recognized by CNN’s shallow layers. Higher-level knowledge of the image is gained via the deeper layers.
The nerve systems seen in biological species serve as an inspiration for neural networks. It is made up of neurons, which act as data processing units, coupled by movable connection weights. The layers of neurons consist of an input layer, one or more hidden layers, and an output layer. There is no set rule that specifies how many concealed layers there should be. The network’s connections between its components serve as a major foundation for determining the function. Each neuron in the input layer is assigned to a certain input parameter. The neural network learns by using a back-propagation method that computes a prediction error by comparing the neural network’s simulated output values to the actual values. The network then back propagates the error through itself, adjusting the weights that are most responsible for the error in an effort to reduce the prediction error. The network is trained or taught by having cycles of data patterns (also known as epochs or iterations) introduced to it. The propensity of the network to memorize the training data following a prolonged learning period is one issue with neural network training. It is more challenging for the network to generalize to a data set that it was not exposed to during training if it overlearns the training data. As a result, it’s standard procedure to split the data set into a learning data set and a validation data set. The learning data set is used to train the network, while the validation data set is used to test network performance.
2.4 Deep Learning for Medical Diagnoses
Deep learning algorithms have improved the speed and accuracy of medical diagnoses in clinics. For the purpose of diagnosing diseases in a healthcare setting, several researchers are creating computer-aided diagnosis systems. The diagnosis of medical signals using deep learning techniques is sole purpose of the research. However, electrocardiogram (ECG) and electroencephalogram (EEG) diagnosis typically employ machine learning techniques (Thiagarajan et al., 2020). To reduce artifact noise and recover the signals’ characteristics, these signals must first undergo pre-processing. The support vector machine, fuzzy neural network, K-nearest neighbor and Adaboost are a few examples of the machine learning techniques that may be used. In fact, machine learning techniques enable faster data processing. However, the qualities of retrieved features and signal processing techniques have a direct impact on machine learning performance.
Recent years have seen the development of deep learning algorithms that can analyze time series signals from devices like gyroscopes and accelerometers for body activity detection, ECGs for the classification of heartbeat arrhythmias, and EEGs for the detection of brain waves. Long short-term memory (LSTM), a sequential deep learning model, and a variant of the recurrent neural network (RNN) have the best performances among the several deep learning techniques for processing temporal information (Liu et al., 2020). Some studies convert the signal’s pattern into an image and perform classification using a two-dimensional convolutional neural network (2D CNN), such as when categorizing arrhythmia in ECG signals and the photoplethysmography-measured pulse waveform signal quality (PPG). A meta-analysis can assess the functional variation of some organs by looking at the transitory response of physiological indicators. (Han et al., 2016) assessed the flexibility of the radial artery using the spectral harmonic energy of the pulse signal ratio. They discovered that palpitation patients had an excessive drop in the spectral energy in the fourth to sixth harmonics. Numerous studies have revealed a strong correlation between the heart rate recovery following exercise and the risk of death. It is an intriguing challenge that calls for new techniques to determine how to apply deep learning algorithms to assess or predict the risks of specific diseases.
2.5 Visual Computing in Healthcare
An area of engineering called computer vision is focused on employing algorithms like CNN to analyze images. Segmentation, detection, and classification are the three primary computer vision tasks. The labeling of a whole image is known as classification. The localization of a specific object within the image is called detection. Segmentation is the process of pixel-by-pixel delineating an individual object’s borders in an image. (Ma et al., 2022) discussed in their study that by the examination of CTPA with PE, these three tasks can be comprehended. It is possible to categorize the entire scan as either pathologic (with PE) or normal (no PE). Additionally, we can find specific emboli. Finally, we can divide the emboli’s pixel-wise bounds.
The conventional test for the diagnosis of DVT is ultrasonography, which is also one of the most accurate methods. More recently, ML approaches have also been used to diagnose DVT. The percentage is not always the same and is initially not very high, but as a sonographer acquires experience and training throughout their career, the tests become more accurate. Nevertheless, there is research that shows promise in combining magnetic resonance imaging (MRI) and deep learning (DL). Artificial neural network analysis has recently been found to improve risk stratification for patients who present with suspected DVT. The authors (Leonardi et al., 2006) demonstrated that a NN is capable of diagnosing DVT without the use of ultrasound and has a low false negative rate. For the quick, unobtrusive, and accurate diagnosis of DVT, a novel ML model was created. This is based on pattern recognition methods that facilitate quick diagnosis as well as properly trained machine learning models that facilitate decision-making and confirm whether a person has this condition or not.
In recent years, the discipline of data science has led the way in the development of both hardware and software for the application of Artificial Neural Networks (ANNs) in clinical diagnosis, which can be useful for the detection of DVT and other disorders generally. ML models include Support Vector Machines (SVM), Decision Trees, and Neural Networks, for instance (Suthaharan, 2016). Alternative DVT diagnosis methods currently exist, some of which use AI (Goodacre et al., 2005). The Random Forest (RF) model, for instance, has superior specificity and sensitivity when compared to the Padua model when used to assess the risk of venous thromboembolism (VTE) in China. By utilizing efficient machine learning to identify the key risk factors of VTE and using patient data from the medical ward at King Chulalongkorn Memorial Hospital in Thailand, the authors developed an automatic diagnosis model. Other initiatives are being made to use ML techniques to predict VTE in young and middle-aged inpatients (Liu et al., 2021); for instance, VTE risk classifiers are being developed utilizing models based on multi-kernel learning and random optimization. These systems’ disadvantages include cost, size, weight, and modest energy usage. On the other side, edge computing can speed up data processing, assure data security (since it is closer to end users, it provides better privacy), cut reaction times, make designs simple, and cost little. It provides good application value and enhances overall data quality and usage efficiency as a result of efficient handling. Benefits include low latency, high dependability, superior energy conservation, and high real-time processing.
2.6 Pulmonary Embolism Diagnosis using Deep Learning
Blood coagulates, causing blood clots to develop. A thrombus is a blood clot that develops in a blood artery or the heart and remains there. An embolus/embolism is a blood clot that exits the site of its creation (disorder). A blood vessel may become attached to by thrombi or emboli, which may then fully or partially stop the flow of blood through the channel. Ischemia is a blockage that hinders correct oxygen intake and regular blood flow. The tissues in that location suffer injury or death (infarction or necrosis) if prompt treatment is not given. Deep venous thrombosis is one of the most widespread types of blood clot problems.
A range of diseases that may appear in various body parts can be caused by thrombi. A number of risk factors, such as prolonged sitting, blood coagulation disorders, inactivity brought on by recent injury or surgery pregnancy, or recent birth complications, oral contraceptive use, estrogen therapeutic, cancer, obesity, stroke smoking, and central venous catheters, increase the likelihood of getting one of these disorders as a result of a blood clot (used for injection of medications or for imaging). Blood clots can result in a wide variety of various ailments, and each disorder has its own unique set of symptoms.
When a blood clot is detected, doctors have access to a wide range of diagnostic methods. These comprise, but are not restricted to, venography, computed tomography scans, duplex ultrasound, magnetic resonance imaging, magnetic resonance angiography, D-Dimer test, arteriography/angiography, and impedance plethysmography. These procedures differ in the approach they take to determining whether a blood clot is present, and they are furthermore made to identify particular diseases.
2.7 Visual Computing for Pulmonary Embolism
Due to free-text narration, medical imaging reports contain significant diagnostic information that is inaccessible to machine analysis. If this unstructured free text was translated into a computer-manageable format, it may be utilised in a number of circumstances needing automatic information extraction. In a recent study, Banerjee and colleagues investigated a deep learning strategy for classifying free-text radiology reports associated with the PE diagnosis—PE present/absent; PE acute/chronic; PE central/subsegmental alone (Chen et al., 2018). They demonstrated great fidelity compared to the most advanced rule-based system and found it to be applicable across institutions for the clinically relevant categories associated with PE diagnosis (Tajbakhsh, Gotway & Liang, 2015).
In another work by the same group (Banerjee et al., 2018), the performance of a deep learning CNN model was compared to that of a classical natural language processing model for extracting PE findings from two institutions’ thoracic CT reports. In contrast-enhanced chest CT reports, the CNN model achieved an accuracy of 99.9% and an area under the curve of 0.97 for determining the presence of PE. As highlighted by these authors, these techniques may make the valuable diagnostic information in radiology text accessible on a large scale for use in models that evaluate imaging utilisation, as part of clinical decision support models, to predict outcomes, and as a valuable tool for evaluating ordering provider imaging yield rates.
Classification of lung vessels as arterial or venous may be of great use to physicians in accurately diagnosing pulmonary illnesses that may affect either the arterial or venous trees. Recent studies have indicated, for instance, that A/V classification allows for a more accurate assessment of pulmonary emboli, whereas arterial tree modifications have been linked to the development of chronic thromboembolic pulmonary hypertension (CTEPH). Additionally, intraparenchymal pulmonary artery alterations have been linked to right ventricular dysfunction. A fundamental method for separating the 2 vascular trunks involves the manual analysis of individual CT slices to trace the blood vessels back to their origin in search of characteristics that distinguish arteries and veins. However, CT picture characteristics such as a large number of slices, scan resolution, and the partial volume effect, as well as the extreme complexity and density of the vascular tree, make this manual separation a time-consuming and error-prone process (Ma et al., 2022). Therefore, the capacity to semi-automatically segment vascular structures on CT images may be essential for enhancing the physician’s ability to diagnose pathological situations (Nardelli et al., 2018).
References
Banerjee, I., Chen, M. C., Lungren, M. P., & Rubin, D. L. (2018). Radiology report annotation using intelligent word embeddings: Applied to multi-institutional chest CT cohort. Journal of biomedical informatics, 77, 11-20.
Batalis, N. I., & Harley, R. A. (2013). Pulmonary Embolic Disorders. Academic Forensic Pathology, 3(4), 420-434.
Bĕlohlávek, J., Dytrych, V., & Linhart, A. (2013). Pulmonary embolism, part I: Epidemiology, risk factors and risk stratification, pathophysiology, clinical presentation, diagnosis and nonthrombotic pulmonary embolism. Experimental & Clinical Cardiology, 18(2), 129.
Brevik, K. A. (2019). Management of pulmonary embolism patients in the emergency department setting (Doctoral dissertation, University of Zagreb. School of Medicine. Department of Internal Medicine).
Chen, M. C., Ball, R. L., Yang, L., Moradzadeh, N., Chapman, B. E., Larson, D. B., … & Lungren, M. P. (2018). Deep learning to classify radiology free-text reports. Radiology, 286(3), 845-852.
Farhat, H., Sakr, G. E., & Kilany, R. (2020). Deep learning applications in pulmonary medical imaging: recent updates and insights on COVID-19. Machine vision and applications, 31(6), 1-42.
Goodacre, S., Sampson, F., Thomas, S., van Beek, E., & Sutton, A. (2005). Systematic review and meta-analysis of the diagnostic accuracy of ultrasonography for deep vein thrombosis. BMC medical imaging, 5(1), 1-13.
Guo, Y., Liu, Y., Georgiou, T., & Lew, M. S. (2018). A review of semantic segmentation using deep neural networks. International journal of multimedia information retrieval, 7(2), 87-93.
Han, X., Chen, X., Tang, X., Chen, Y. L., Liu, J. H., & Shen, Q. D. (2016). Flexible polymer transducers for dynamic recognizing physiological signals. Advanced Functional Materials, 26(21), 3640-3648.
Leonardi, M. J., McGory, M. L., & Ko, C. Y. (2006). The rate of bleeding complications after pharmacologic deep venous thrombosis prophylaxis: a systematic review of 33 randomized controlled trials. Archives of Surgery, 141(8), 790-799.
Liu, H., Yuan, H., Wang, Y., Huang, W., Xue, H., & Zhang, X. (2021). Prediction of venous thromboembolism with machine learning techniques in young-middle-aged inpatients. Scientific Reports, 11(1), 1-12.
Liu, W., Liu, M., Guo, X., Zhang, P., Zhang, L., Zhang, R., … & Xie, S. (2020). Evaluation of acute pulmonary embolism and clot burden on CTPA with deep learning. European radiology, 30(6), 3567-3575.
Ma, X., Ferguson, E. C., Jiang, X., Savitz, S. I., & Shams, S. (2022). A multitask deep learning approach for pulmonary embolism detection and identification. Scientific Reports, 12(1), 1-11.
Müller, D., & Kramer, F. (2021). MIScnn: a framework for medical image segmentation with convolutional neural networks and deep learning. BMC medical imaging, 21(1), 1-11.
Nardelli, P., Jimenez-Carretero, D., Bermejo-Pelaez, D., Washko, G. R., Rahaghi, F. N., Ledesma-Carbayo, M. J., & Estépar, R. S. J. (2018). Pulmonary artery–vein classification in CT images using deep learning. IEEE transactions on medical imaging, 37(11), 2428-2440.
Shen, D., Wu, G., & Suk, H. I. (2017). Deep learning in medical image analysis. Annual review of biomedical engineering, 19, 221.
Suthaharan, S. (2016). Machine learning models and algorithms for big data classification. Integr. Ser. Inf. Syst, 36, 1-12.
Thiagarajan, J. J., Rajan, D., Katoch, S., & Spanias, A. (2020). DDxNet: a deep learning model for automatic interpretation of electronic health records, electrocardiograms and electroencephalograms. Scientific reports, 10(1), 1-11.

Get expert help for Blood Clot Detection In Lungs and many more. 24X7 help, plag free solution. Order online now!