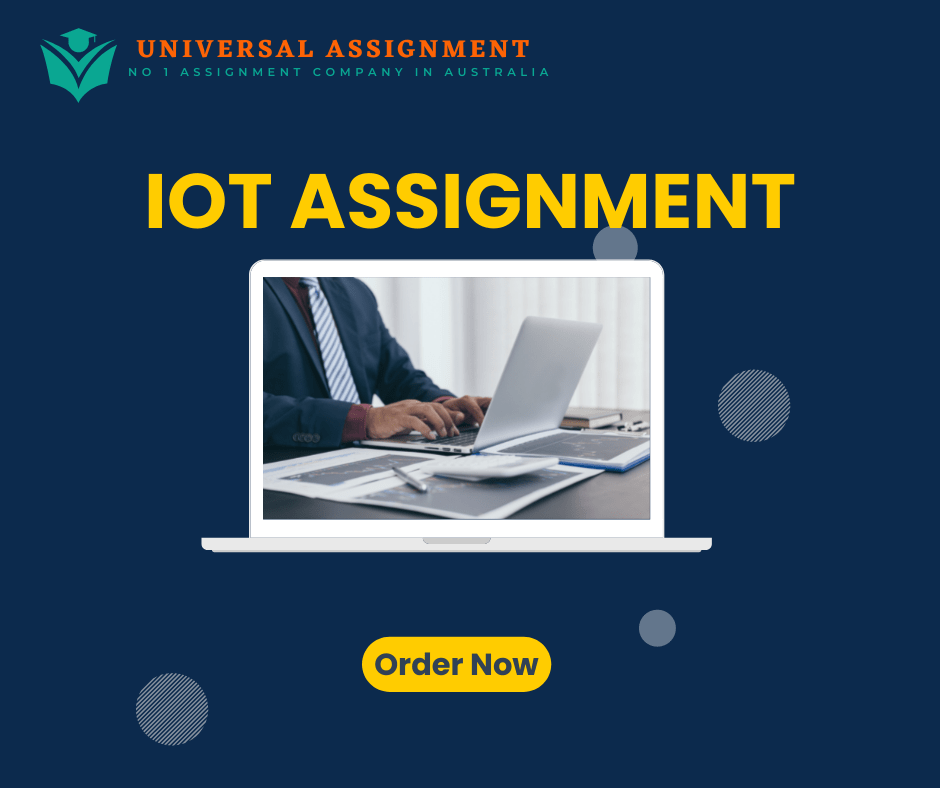
DATA ANALYSIS OF CLOTHING INDUSTRY FEEDBACKS
EXECUTIVE SUMMARY
In this report, we have explained the women’s clothing industry different content and then analyzed data based on reviews of different clients. Under analysis, the different age groups were involved and the ratings associated through the tabular form data were analyzed to gain optimal results. Attributes in the table were age, reviews, ratings, department of clothes with enough. For future product design, sales predictions, trendsetting, product suggestions, tailored service design, and decision-making, consumers’ projected interests and needs, and customer feelings about brands and goods may be employed. Companies must be able to gain useful insights from current data and apply such findings to working practices to enhance their competitiveness and flourish in a world rich with information.
Further, we have explained the background of the data to collect it. Along with that, the terms and terminologies were explained that were inherited for gaining the desired figures for analyzing accordingly and the future scope explained with the recommendations that can help in enhancing the productivity and organization too.
TABLE OF CONTENT
Introduction……………………………………………………………………………. 3
DATA ANALYSIS……………….…………………………………………………………………… 5
Conclusion……………………………………………………………………………………………. 9
References……………………………………………………………………………… 10
INTRODUCTION
Continuing to digitalize company activities and fashion businesses are no exception. By developing mobile devices, the Internet, SNS services, and a host of other new data-creative technologies, fashion firms have easy access to a variety of new sources for information such as click rates on the website, browsing history, and website reviews and comments on social media. Many of these data are relevant for the fashion industry. In the fashion business, data analyses are both significant in the past and today.
For fashion sales predictions several sorts of statistical techniques have been used, such as time series analysis, regression analysis, and multiple factor analysis done concerning data for understanding the continuous difference between sales and profits.
Data visualization provides an accurate understanding of what the information means by creating a mapping or graphic visual context. This helps the human mind to understand the data more naturally and so facilitates the identification of trends, patterns, and outliers in broad collections of data. Data visualization enables us to grasp information quickly and clearly. Thanks to graphic representations, enormous amounts of data are shown in a comprehensible and cohesive manner, which in turn enables us to interpret information and learn from it. The fundamental objective of data visualization is to communicate information swiftly, attractively, correctly, and sensibly. It only accomplishes its goals if it codes the data such that our eyes can identify and understand our brain. Visualization is successful because it uses our subconscious mind’s ability.
Python may be applied well beyond entry-level programming although it is easy to learn. It is utilized at the highest data analysis levels routinely. That is why when you create most of our data visualization applications, Python is the language of choice (Waskom, 2021). A way of filtering data frames is utilized for pandas isin(). isin() helps pick rows with a specific value in a column. The isin() function is helpful. Parameters: The values of the caller’s series/data frame are: Iterable, series, list, tuple, data frame, or dictionary for verification. Data visualization means the graphical image of the data to transmit insights to consumers, customers, and stakeholders in general interactively and efficiently. Matplotlib (Chen et al, 2020). The most used Python library for viewing information is undoubtedly Matplotlib. Everyone interested in data science undoubtedly at least once used Matplotlib. matplotlib. Pyplot is a collection of matplotlib utilities such as MATLAB. Each pyplot method changes certain figures: for instance, the plot is created, a trace area is created, lines are trained, the plot is decorated with labels, etc.
Pandas was one of the most prominent and popular data science tools for data wrangling and analysis used in the Python programming language. And when cleaning, converting, manipulating, and analyzing data Pandas is truly a game-changer. Pandas help to purify the chaos in simple words.
Today many research studies focus on social media, especially as this covers the majority of the world’s population; a large number of individuals have access to mobile devices and also social media services users. Social networking is an excellent resource to use BDA (Jimenez-Marquez et al, 2019). Preferences, exploring everyday trends, understanding associated user behavior, or analysing demographic habits. Social media have the data needed to assess individual product reviews
A dataFrame represents a table of data with columns and rows as the most popular Structured API. The column list and the types in the schema columns. An analog with named columns would be a spreadsheet. Pandas DataFrame has a double-dimensional, possibly heterogeneous, labeled axis tabular data structure (rows and columns). A data frame is a two-dimensional data structure, i.e. data in rows and columns is aligned tabularly. Pandas DataFrame has a double-dimensional, possibly heterogeneous, labeled axis tabular data structure (rows and columns). A data frame is a two-dimensional data structure, i.e. data in rows and columns is aligned tabularly.
DATA ANALYSIS FINDING
While analyzing data following datatypes were used for proper visualization-
Clothing ID int64
Age int64
Title object
Review Text object
Rating int64
Recommended_IND int64
Positive_Feedback_Count int64
Division_Name object
Department_Name object
Class_Name object
Class object
type: object
Below photo having tuples with count, mean, min, and various optimistic results concerning clothing ID, age, rating. Here we have considered ratings into two terms that are positive and negative.
Positive feedback comes under consideration while taking rating more than or equal to 3 when we are taking the highest value to be given to rating as 5 and Negative feedback as feedback given is less than 3.
The below graphs can help us in understanding the number of good and bad reviews concerning several people through which we have gathered data. As it is clear that several good reviews are more than bad reviews. So, simply this helps us to aware about correct measures to be adapted.
The below graph explains that the age group of 31-50 are mostly unsatisfied with the quality of products provided by the clothing industry.
Below we have visualized the different products with the number of people who buy them.
These outcomes can help us all in making overcome strategies for gaining profits and attracting the age group that needs to be attracted and providing consistency to all buyers in terms and quality and service who are satisfied.
CONCLUSION
Building your reviews creates the chance that a Google search engaged your business when it was found. Try to provide more effective and beneficial services to the client with the best quality to gain better reviews. With that try to provide them discounts and other offers and characterize each consistent customer important or provide them prime membership to make them feel their importance. To allow consumers to submit a review on Google for your company, they must visit Google Maps, search for your company, upload their Google Business Profile, scroll down to the review area, and then click on the review prompt. An explanation on your website is one method for more reviews. Knowledge of this Google is equipped with a feature for establishing a shortcut to the review area of your corporate profile. As the aforementioned technique functions, it is best even to construct a whole site page that can be accessed from your main navigation menu for Google reviews (or reviews, in general). Both a CTA for review but also current reviews should be included on the page. This not only promotes chances of becoming a client but also inspires a current consumer to submit a review. That can be used to make changes for the welfare of the organization.
REFERENCES
Jimenez-Marquez, J. L., Gonzalez-Carrasco, I., Lopez-Cuadrado, J. L., & Ruiz-Mezcua, B. (2019). Towards a big data framework for analyzing social media content. International Journal of Information Management, 44, 1-12.
Oelke, D., Hao, M., Rohrdantz, C., Keim, D. A., Dayal, U., Haug, L. E., & Janetzko, H. (2009, October). Visual opinion analysis of customer feedback data. In 2009 IEEE symposium on visual analytics science and technology (pp. 187-194). IEEE.
Fahad, S. A., & Yahya, A. E. (2018, July). Big Data Visualization: Allotting by R and Python with GUI Tools. In 2018 International Conference on Smart Computing and Electronic Enterprise (ICSCEE) (pp. 1-8). IEEE.
Waskom, M. L. (2021). Seaborn: statistical data visualization. Journal of Open Source Software, 6(60), 3021.
Cordeil, M., Cunningham, A., Dwyer, T., Thomas, B. H., & Marriott, K. (2017, October). Imaxes: Immersive axes as embodied affordances for interactive multivariate data visualisation. In Proceedings of the 30th Annual ACM Symposium on User Interface Software and Technology (pp. 71-83).
Chen, H., Zheng, G., & Ji, Y. (2020). Generating hierarchical explanations on text classification via feature interaction detection. arXiv preprint arXiv:2004.02015.

Get a fresh solution by the industry experts for DATA ANALYSIS OF CLOTHING INDUSTRY FEEDBACKS Assignment and many more. 24X7 help, plag free solution. Order online now!